Part 3: When and where is AI ethics needed?
It's nice to know that AI ethics is a must when your organization is deploying AI to solve societal problems or better serve the public. Simply putting in the money won't take you very far. Making AI work for society is the work of people after all. But how to do it then? When and where should ethical reflection on AI be introduced in public organizations?
In this final part of the introduction series on "AI ethics for public organizations," the separate phases of AI usage are distinguished. They are related to helpful ethical questions that are specific to those phases, and we share insights on where to situate the AI ethics capability in your organization.
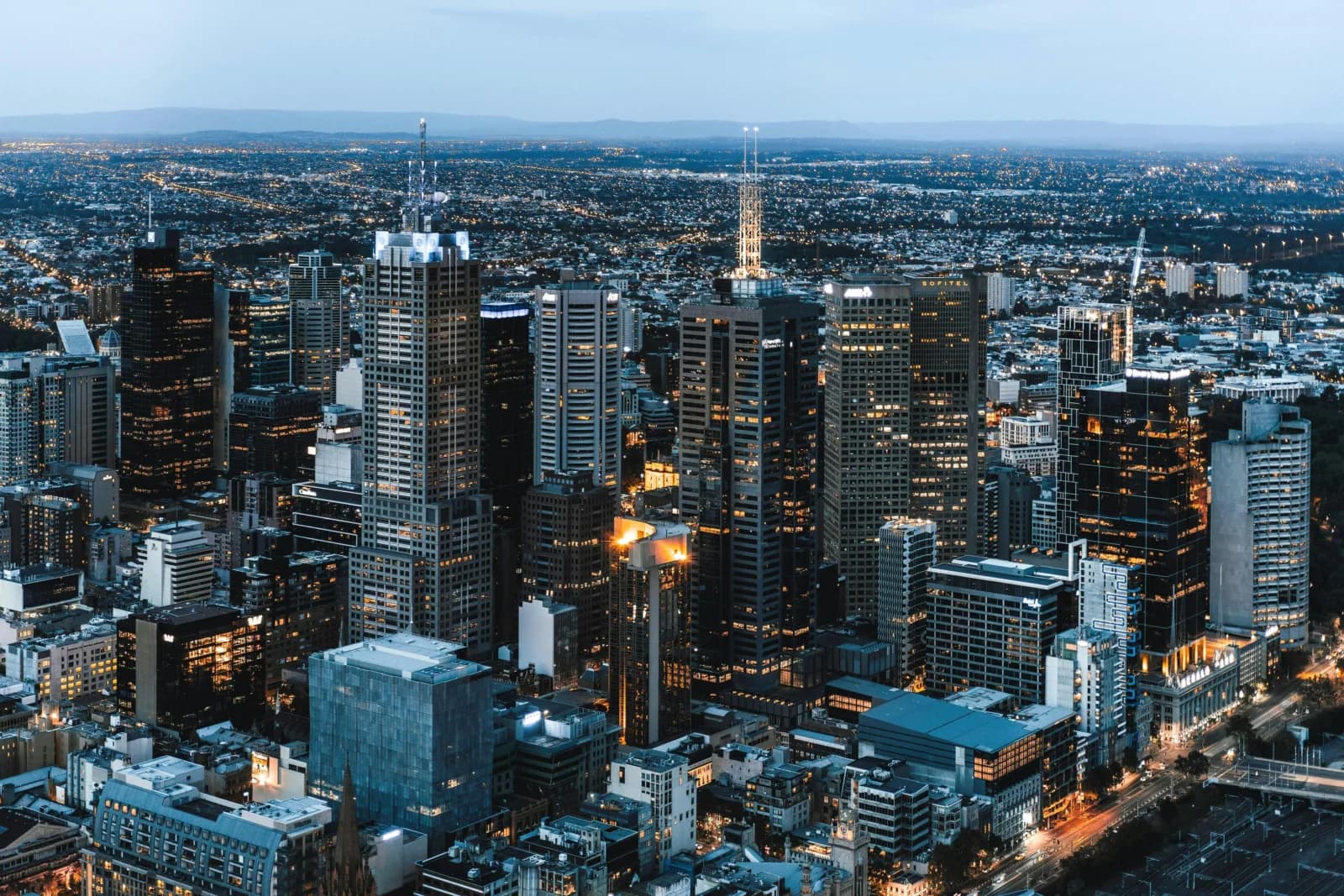
When to practice AI ethics?
So, when should we practice AI ethics in an organization? The short answer: In all phases of AI development and deployment. To unpack this apparent cliché, it is helpful to view the role of AI ethics in the different phases of AI systems. We discuss each of these phases in turn.
- In the design phase, the relevant stakeholders come together to determine the use case and the type of AI that is needed to fulfill the business demand. After obtaining legal compliance, ethical reflection involves the inclusion of the right stakeholders, describing the use case and what organizational and public values the system will touch upon. It also involves designing the desired workings of the production phase and the interactions with human users. Relevant ethical questions in this phase are: Who will the system affect? What happens when the system malfunctions? What societal goals are in scope for the system to contribute to?
- Next, in the data collection phase, existing datasets are selected. Or the necessary data are specified, gathered, and possibly further annotated. Whose moral perspective is ‘baked’ into the data, that is: How data are defined, and who gathers and annotates, are important ethical questions to consider in this phase.
- In the subsequent training and testing phase, the AI model is tuned towards adequate performance on the specified use case. This is done according to some metrics, such as accuracy for classification. The tuning of modelling parameters happens in this phase, but it is also important to set clear requirements for model performance based on the use case from the design phase. For example: If the output of the AI system leads to a decision, what margin of error is acceptable? What do we do when the system malfunctions and who is accountable and/or able to respond adequately? These are questions that should be spelled out so that the training and testing phase can take them into consideration.
- The production phase is where the AI system is in deployment, and humans act together with or on the system’s output. Here ethical reflection is tailored to monitoring performance and putting checks and controls for the system in place so that the AI system works as expected. The remaining ethical risks need to be mitigated without introducing complications or non-transparent decisions.
- Finally, the evaluation phase runs parallel to the production phase and involves periodically questioning whether the AI system still meets the business demands and design requirements. This can take the form of human rights impact assessments, algorithm audits or AI risk management systems.
Where should AI ethics be situated in an organization?
Next, we can ask: Where – in what role – should the responsibility for ethical reflection be allocated? Recent research has highlighted that ‘the latent space of data ethics’ is where reflection on algorithmic risks and benefits can be incorporated. Data ethics is the addressing of moral challenges concerning everything that has to do with data. AI ethics can be seen as a subfield of data ethics.1
Building the ethics layer
The best space to allocate this capability is the so-called ‘ethics layer’ of an organization. It is positioned between the legal layer concerned with compliance with law and standards and the executive layer for data and AI governance. When risks with respect to data and algorithms are concretely defined, the ethical questions ideally land with a ‘chief data ethics officer’ or ‘AI ethics officer’. Less ideally, they will be allocated with the chief data officer or even with business-level data workers. In absence of an AI ethics officer, some propose the introduction of committees for algorithmic risks or data ethics to better connect the executive and legal layers around the core of data operations. It is important to consider these options because the benefit of practicing ethics in this space is that it enables identification of the ethical gaps left open after AI operations satisfy legal constraints, such as the AI Act. The questions we asked before like ‘what stakeholders are affected and how?’ and ‘what public or organizational values does this AI system target?’ remain open but need to be filled in and translated from data or AI problems to the executive level.
But there is also a clear role for ethics officers at the conception of an AI system. Namely in advising the executive layer on the question: What do we actually want to achieve and why?
Getting AI ethics off the ground
With the basics, necessity and ‘when and where’ of AI ethics explained, it is time to put AI ethics to work. But enacting AI ethics is demanding and difficult to establish continuously for most public organizations. Sadly, it is therefore ignored or forgotten. AI ethics is also something that your organization can lack the capability to do. This is where Highberg can help you out. We have experience setting up algorithmic committees and supplying ethics officers. We provide hands-on and lasting implementation of data and AI ethics in your organization and are able to fulfill internal roles ranging from the governance, ethical and executive layers.
This series of primers will be followed by a position paper on AI ethics that presents a risk-based approach public organizations can place at their disposal to implement ethical processes in their AI design, development, and deployment.
Interested in what we can do for you, or do you have thoughts or use cases to share? Feel free to contact our consultants.
References
- Enrico Panai, “The latent space of data ethics,” AI & Society (2023): 1-15.
Related Insights
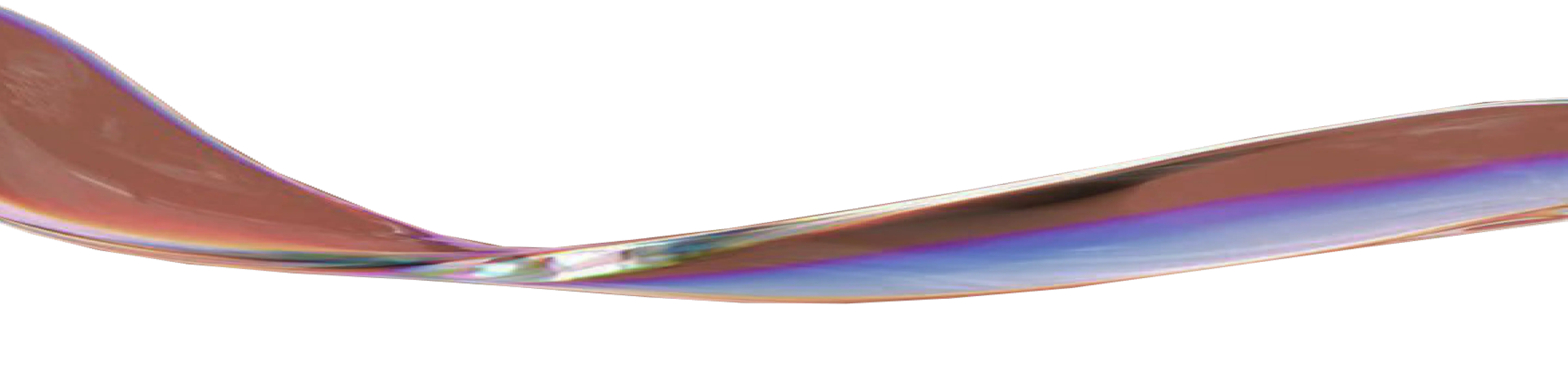