The hub and the spokes: how do we organize our data expertise?
How do we get more out of our data? We ask our data specialists, but where are they in our organization? In a separate corner behind big screens and fat computers, among employees in departments or somewhere in between?
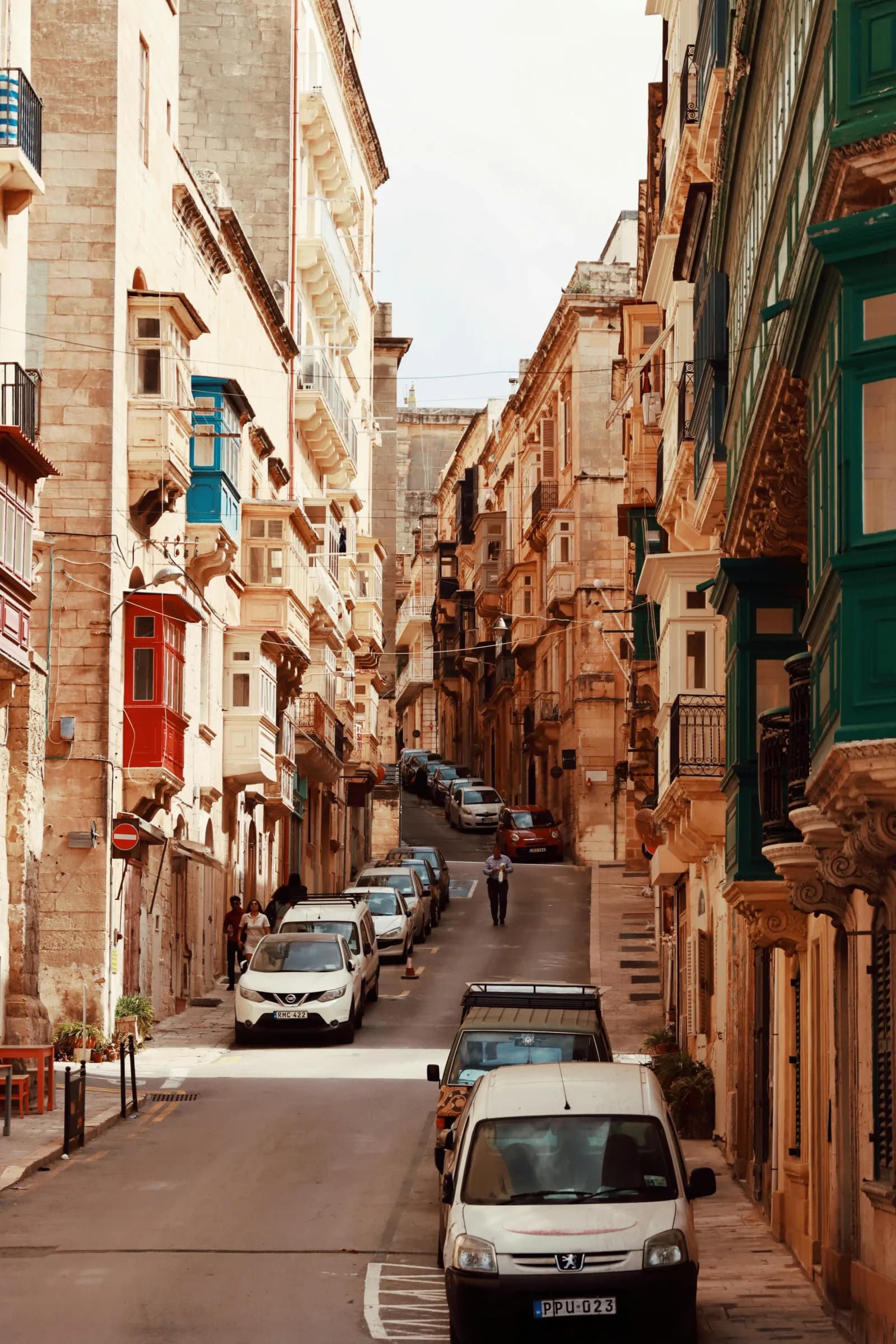
The data organization issue
Many government organizations are grappling with this issue. Responsible data use is becoming increasingly important. We are hiring data specialists - which is often already a tall order - and more employees are going to have data competencies. Because they like it and because they see that data helps solve social issues.
In this blog, we address the organizational issue created by the arrival of data specialists and the increasing importance of data in the execution of public tasks
The protagonists
Who do we all meet here? First, the employee who wants to "do more with data" in his/her department. Let's call her Nuria the data translator. She recognizes the data demand in her department and has an idea about the possible contribution data can make to work. In addition, Menno enters the stage. Menno was brought in as a data scientist because we need more data expertise in our organization to answer questions like Nuria's. He can build data solutions, help with analysis and knows which technology is helpful for which issue. We also have Julia, who as a data engineer was tasked with unlocking new datasets in the data platform (the IT tooling for storing, analyzing and using data). Finally, we have Karel. Karel is the manager to lead the data team in formation. Karel is tasked with setting up the data team in the most effective way and giving it a strong position within the entire organization. What are his options in this regard?
The extremes
We set up a central data team with its own people and its own responsibilities. Nuria, Menno and Julia, together with the other data specialists, are placed in a team of which Karel becomes the manager. Employees from the departments can come to the central data team with their data questions and the data team will work on them.
Handy to have all these specialists together. In the data team, a lot of knowledge in the field of data is created and the data specialists can work in a standardized way. There is also clarity about the management of the data and the data products: this lies with the central data team. As a manager, Karel is well informed about what is going on within 'his' team and can cleverly allocate tasks.
A central data team often works well for small organizations: the lines of communication are short, making it relatively easy to find the central team. Also, organizations where data maturity is low can quickly create sufficient scale and take the first steps with data-driven work with a central team.
What is inconvenient about a central data team, however, is that departments do not always ask clear data questions. Because Nuria, Menno and Julia work centrally in the organization, they have relatively little contact with people from the departments and do not know what is going on there. They are not always able to clarify the data questions from the departments. As a result, the data team does sometimes go down its own stubborn data road. Somehow, they in the departments are far from always satisfied with the data products the data team comes up with.
There is an alternative: the data specialists work completely decentralized. Each department has its own data specialists. Nuria can (fortunately, she thinks) stay in her own department and work on data projects there. Menno and Julia are added to her department as data specialists. There is no room for Karel for a while so he has to do something else. After all, there is not just one data team, so it is impossible to manage.
Handy, those data specialists so close to the primary process in the departments. No need to set up a new department.
A decentralized model can work well when an organization has different 'speeds' in the field of data-driven work: a certain department wants to work faster than the others and thus has the freedom to realize its ambitions with its own data specialists. Even in very large organizations in which departments are very independent and have little or nothing to do with each other, a decentralized model can work well.
What is awkward about the decentralized model is that it hinders the further development of the data knowledge of the data specialists. Moreover, each department does go its own way and efficient standardized solutions are out of the question. Who is responsible for proper management of data and data products? And what do we do with data issues that transcend departmental boundaries?
The third way: hub and spoke.
There is another model. Suppose we set up the organization as follows: we establish a common data team where Menno, Juliet and and the other data specialists provide their services to the departments under the direction of Karel. The data specialists have one leg in the central data team and one leg in the department(s) for which they develop data products. This team is the "hub," the hub in the bicycle wheel.
Nuria, our data translator, just stays in her department. The other departments also have data translators who, with their combination of subject knowledge and data expertise, help colleagues in the departments make good data inquiries. They are in close contact with the data specialists and know what solutions they can provide to their departments. They are the "spokes," the spokes in the bicycle wheel that are firmly anchored in the hub and form the connection to the wheel. The place where meters are made.
This is handy, because the spokes receive a lot of knowledge from the hub about the possibilities and impossibilities of data for their work, and vice versa, the hub receives a lot of information via the spokes about what is going on in the departments. As a result, the data team does not become disconnected from the departments. Data projects are more likely to be used efficiently and responsibly. The joint data team can also help the entire organization develop and implement a data strategy. Especially if Karel, our manager, acquires a strong position in the management of the organization. Together, the hub and the spokes make a strong wheel. Awkward may be the complexity: how is the cooperation between the central team and the departments? What is the balance between autonomy for the departments and conforming to joint agreements?
Each model has its advantages and disadvantages. The size of the organization, the "data maturity" and the organizational culture (including the degree of autonomy of the departments) determine the choice of one of the models. For more information about an effective and efficient data organization contact Highberg at gijs.degroot@Highberg.com in particular.