Part 2: AI ethics: mitigate risks and harness potential
If you want to apply AI to your organization's problems, where do you start? How do you do so in line with the public values you want to protect? Like all technologies, whether AI helps or harms depends on how you use it. But ignorant use of AI is more likely to do harm than do good. This means not reflecting on how AI relates to your organization's goals and values. 'Why is AI ethics necessary for every organization using AI systems?' is therefore the second question this series of introductions on "AI ethics for public organizations" addresses.
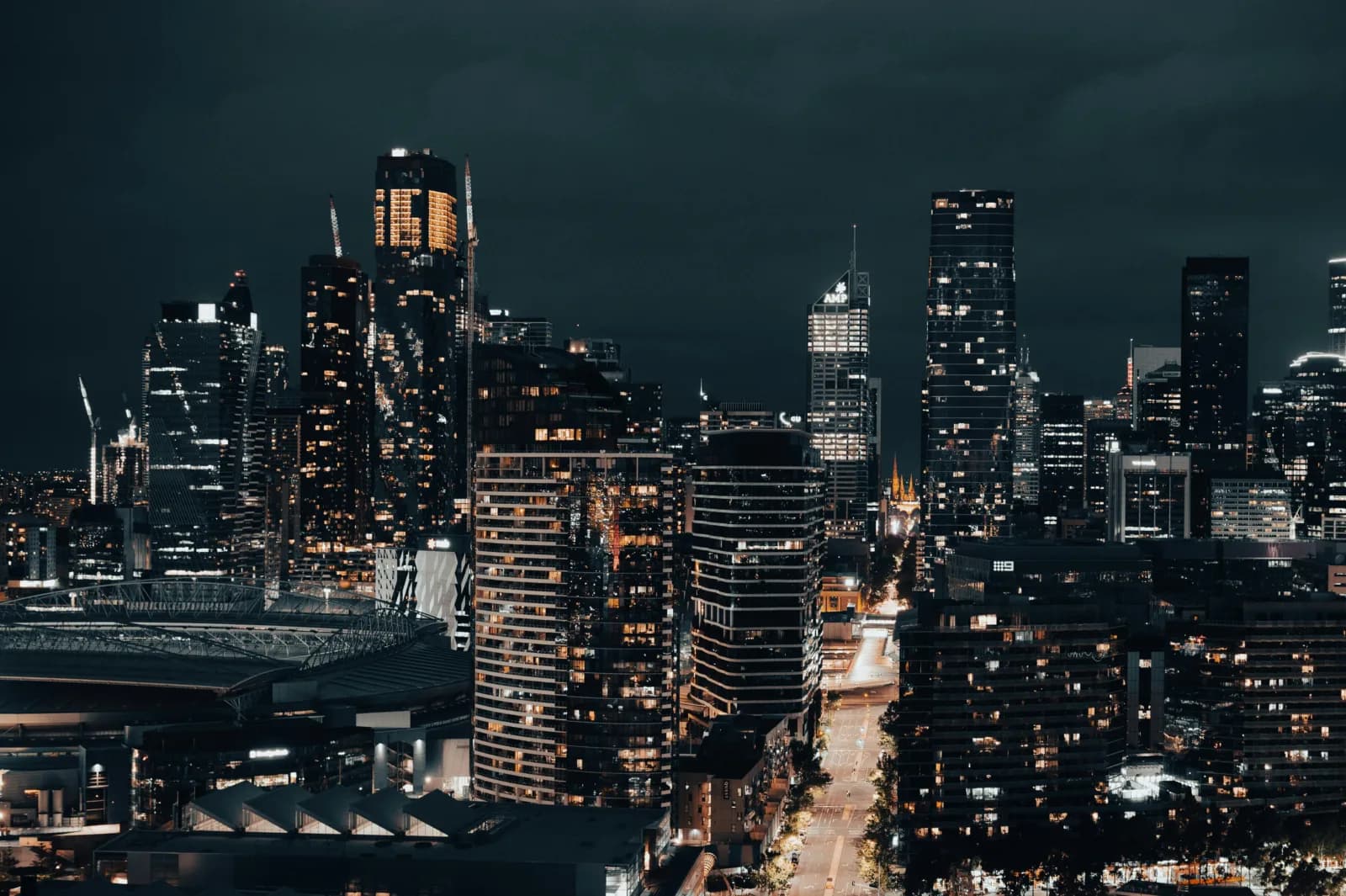
Centralizing ethical risk
The concept of ‘ethical risk’ is our first step towards establishing the necessity of AI ethics. ‘Risk’ is the combination of the gravity of a harm and how likely it is to occur.1 And ‘ethical risk’ is defined as risk concerning an AI system that can lead to stakeholders in the system's failure of ethical responsibilities towards other stakeholders.2
Because these definitions are still broad and a little vague, we illustrate them using three simple examples that are acceptable non-high-risk cases under the AI Act: (1) algorithmic bias, (2) untrustworthy AI and (3) misalignment.
In the ethics of AI, we should move from reflection on the moral context of the system to risk mitigation by implementing measures for when risks are expected to occur. The examples show how identifying and addressing ethical challenges is of the essence for both preventing unwanted consequences and harnessing AI’s potential. Identifying the ethical risks and mitigating them is therefore at the core of putting AI ethics in practice.
Algorithmic bias (1)
Machine learning-based AI systems are only capable of providing the right predictions or classifications if the data used to train them is of the right quality and quantity. The problem lies with the way input data are gathered or how the model itself is defined and trained. This has repercussions for the inevitable ‘biases’ the AI system has. It is ethically important to be aware of a system’s biases. Such awareness enables that the ‘false positives or negatives’ an AI system outputs can be tackled by the user interacting with the system. An example is correctly flagging misinformation or an incorrect prediction. If the user is unaware of the specific biases, then flawed outputs are accepted uncritically and acted upon. Furthermore, as a deployer one is accountable for decisions made based on the AI system used. So ethical awareness of algorithmic bias is essential for safe and responsible use of AI systems by public organizations.
Untrustworthy AI (2)
Consider the application of AI systems to managing the logistics of the transportation of people with disabilities to school, work, or care institutions. Some public organizations are tasked with organizing this transport efficiently and function as interface between people with disabilities and the institutions they depend on. In this case, the ethical risk of the AI system is the stakeholder that is harmed when the system malfunctions. In other words, if the algorithmically generated route structurally misses or arrives late at some homes, then the person to be transported bears the burden of the system’s failure. This is an ethical risk – a failure to fulfill a duty – which is intolerable. On the other hand, there is an ethical benefit to be harnessed by optimizing this AI system: Improving and smoothening the access of people with disabilities to institutions like work, school, or care. As such, AI’s potential instantly touches this type of public organization’s core operations.
Misalignment (2)
Finally, there is the problem of ‘aligning’ AI systems. The problem of alignment is as old as automation and robotics: You want machines to do what they were intended to do. However, in the case of AI systems this problem takes on a new guise. Incorporating ‘rules’ for machine learning applications to follow is harder to do than for logic-based expert systems. This is because the question why they output what they do is harder to answer. Their internal workings are often non-transparent. So, when depending on AI systems in decision-making, the ethical risk is that the operations are out of touch with (a) what their users expect of them to do or (b) what the organization’s values are. Misaligned AI thus lead to ethical risks to be mitigated, while aligned AI are what any use case wants to establish and where the ethical benefit of the system lies. In other words: The automation of solving a problem exactly the way it was intended to be solved. Aligning AI systems in their performance with the wants, needs and beliefs of users and organizations is therefore simultaneously both risk mitigation and benefit capture.
Giving AI's ethical risks due attention
Many other examples could be given of ethical risks related to the technical aspects of AI systems. But the takeaway point is that ethical risks and benefits are as diverse as the use cases of AI systems. Ethical reflection is necessary to understand the dangers and opportunities your organization faces when developing and deploying AI. AI ethics shouldn’t be shunned or dismissed: It is essential to establish technology that reaches your organization’s goals and demands.
It may be valuable to know that if you’re on your way with AI ethics but need ethical verification or validation, Highberg can help. We offer AI audits and can support the development of algorithm registries to clearly document ethical aspects of existing AI systems.
For support in the design phase, guidance on Human Rights and Algorithm Impact Assessments might be helpful to free the use case an organization is pursuing of any unintended potentially harmful consequences.
Want to learn more about what we can do to get your AI ethics off the ground? Check out our capability ‘Responsible AI’ or reach out to one of our consultants.
References
- European Commission, “AI Act,” Article 3.2.
- David M. Douglas, Justine Lacey and David Howard, “Ethical risk for AI,” AI and Ethics (2024): 1-15.
Related Insights
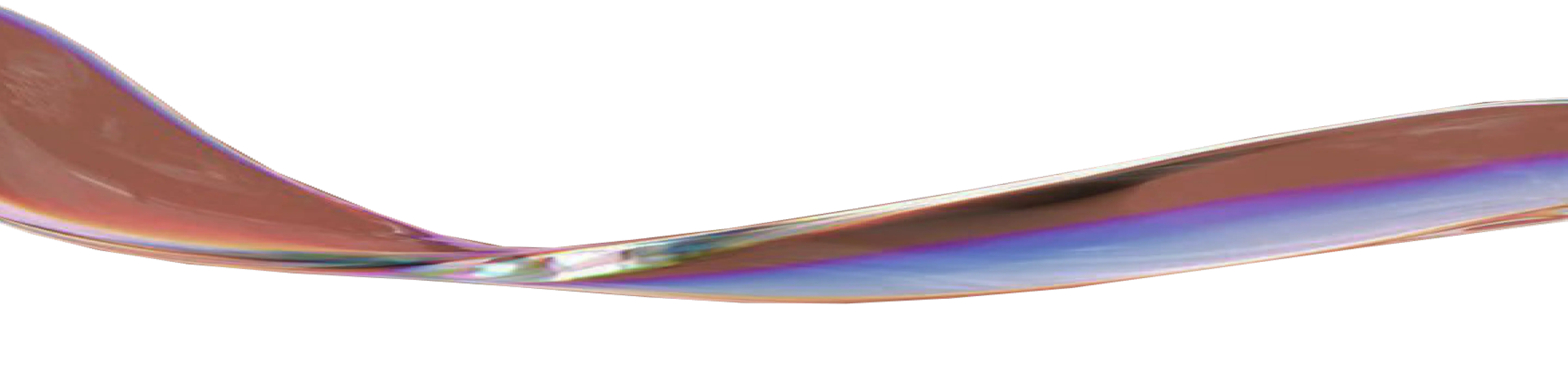