Part 1: The basics of AI ethics
‘What really is the ethics of AI?’ We often get this question from public organizations. Joining AI and ethics might seem strange at first glance, but raising ethical questions is essential to make AI work for your organization. No automating technology is a 'quick fix' for the difficult societal problems that public organizations deal with. Only AI systems that are 'sensible by design', well thought-out, and steer clear of ethical risks succeed in helping solve large, societal challenges. Think of making health care more effective, efficiently sharing information with stakeholders, and strengthening social security and well-being.
The role of ethics in applying AI to societal challenges is therefore worth examining.
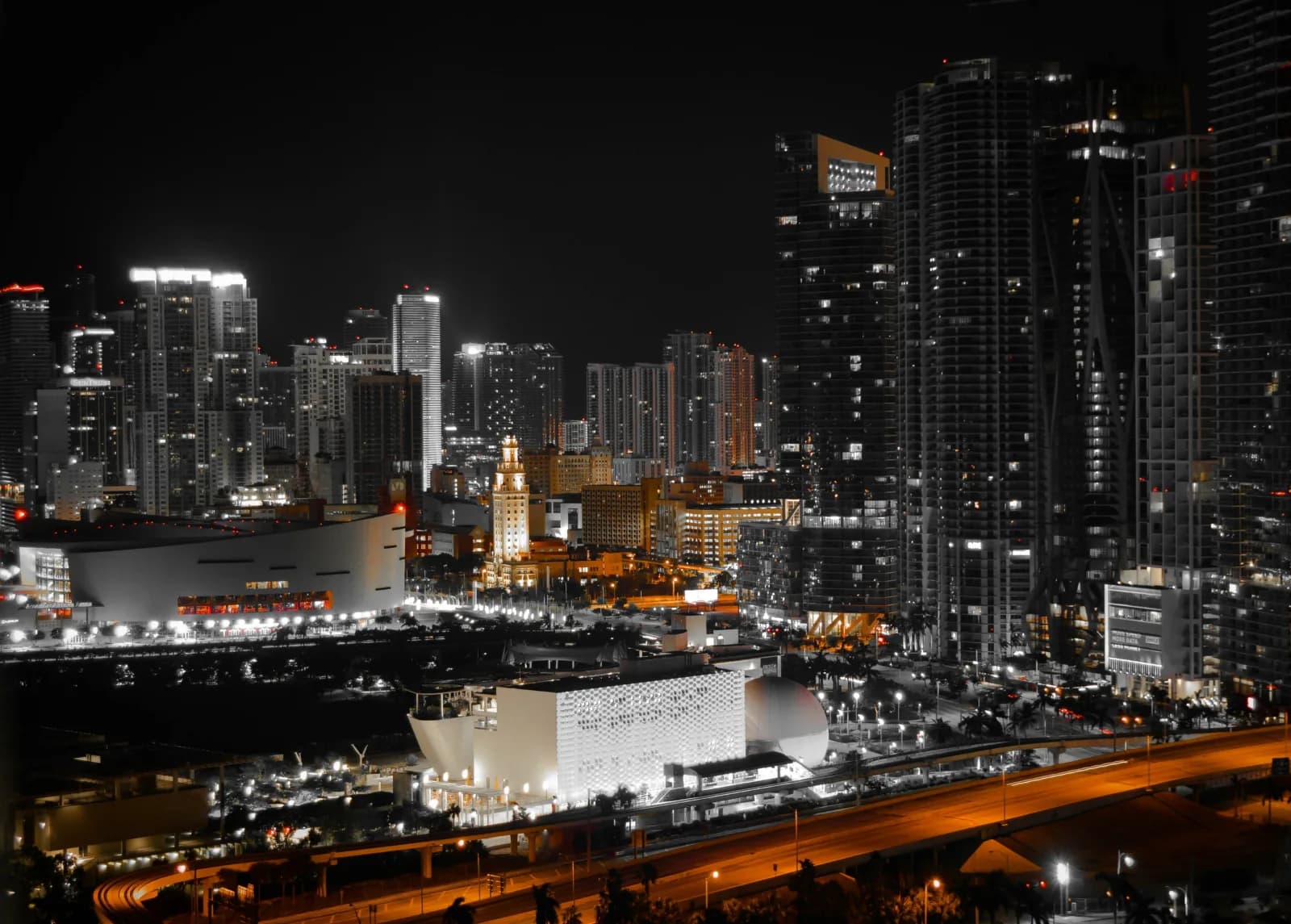
What is AI ethics?
To answer the question of what AI ethics is about, we always start with defining what an AI system is. The EU AI Act's definition is a helpful place to start. In the AI Act, AI systems are defined as “machine-based” and “designed to operate with varying levels of autonomy and [...] may exhibit adaptiveness after deployment”.1 The system produces an output that directly influences its (virtual) environment based on an input it receives. ‘Output’ can here mean the production of content, a prediction, or some automated action. These AI systems, such as expert systems, machine learning, deep learning or foundation models, bring along moral questions, dilemmas, and opportunities. Simply put, AI ethics deals with these challenges.
These challenges are two-sided: For each AI-system, there are harms to be avoided and benefits to be captured. AI ethics is therefore concerned with the societal duties to avoid harm and do good.2
Start with a question
For public organizations, not all ethical challenges regarding AI are in play simultaneously. Ethical reflection on AI applications is always a situated affair. Context matters for AI. As a first step, asking questions about this context is helpful:
- Why do we pursue an AI solution to the problem we have?
- What organizational and societal values does the problem that the AI system solves relate to?
- Which stakeholders are affected in the distinct phases of the AI system’s development and deployment?
Taking these questions seriously underpins what AI ethics for public organizations should be.
To answer these questions, an organization should aim to clear up at least three things. (1) The AI system’s relation to public values; (2) how the AI application is embedded into society; and (3) what conceptual tools are useful for establishing ethical AI. We’ll walk you through each aspect.
(1) AI and societal values
Because of its use case, an AI system relates to certain societal values.4 We should pay close attention to these values because they can be threatened as well as strengthened. For example, in a system that processes sensitive personal information for algorithmic decision-making, related values include privacy, explainability and equity. Alternatively, when machine learning is applied to environmental challenges such as combatting heat stress, managing logistics or saving energy, sustainability, accuracy, and control are values to think well about. Clearly different values demand different ethical questions and resources. But in each case iteratively questioning the choices underlying the AI system and sensitivity to its contextual values are imperative. An example of a design methodology that emphasizes exactly this is ‘value sensitive algorithm design,’ or VSAD for short.3 In VSAD, documenting the values of the stakeholders involved in detail underpins the complete design process.
(2) AI’s relation to society
Every AI application is a sociotechnical system.4 AI is always embedded into the social role of the organization deploying it. An AI system’s ‘ethical profile’ is supplied by the actors involved in its design and who interact with it in the deployment phase. It is therefore important to recognize what stakeholders have contributed to the AI system, as opposed to the stakeholders that will be affected by the AI system. A helpful way to think about this is via the paradigm of ‘participatory AI.’ This perspective emphasizes the need for participation. It prescribes that the affected stakeholders also need to be involved in the design of and discussion about the AI system or should at least have been adequately consulted.5 In the case of public organizations operating in the social domain, stakeholder involvement is the central ethical concern. For example, stakeholder involvement can minimize algorithmic bias in the data gathering phases by introducing stakeholders in the data curation process. It can also positively affect the design phase by introducing them in the process of determining the right classificatory outputs for the system. Likewise, if stakeholders are subjected to the output of an AI system, they should be involved in the evaluation of the system too.
(3) Tools for practicing AI ethics
Lastly, it is important to know what is in the toolbox for realizing AI ethics. Different ethical perspectives are ‘the conceptual tools’ with which one practices ethics. These perspectives are often complementary and highlight aspects of the same ‘good’ or ‘bad’ usage of a system.6 For example, one could think of what usages of the system in production one wants to cultivate or take precautions against. This is complementary with asking how the system can strengthen stakeholder-interrelations or threaten to affect these relations negatively. Similarly, one could make harm/benefits analyses of the system’s consequences or think about users, developers and deployers' rights and duties to highlight yet other important ethical aspects.
Okay, so I understand what AI ethics is: What now?
Do you feel that you lack the resources and know-how to realize AI ethics? Achieving insight into AI systems’ relations to societal values and stakeholders faces a competence barrier and can be time-consuming. Highberg provides the on-demand deployment of an AI ethics officer to help apply this capability on a continuous basis.
In the next part, we show why practicing AI ethics is a ‘must have’ rather than a ‘could have.’ We clarify how to balance risk mitigation with reaping AI's benefits. Can't you risk delaying the implementation of AI ethics? Reach out to our AI ethics team to start with the ‘why, how, where and when’ of AI ethics today.
References
- Europese Commissie, “AI Act,” Article 3.1.
- Sven Nyholm, “What Is This Thing Called the Ethics of AI and What Calls for It,” in Handbook on the Ethics of Artificial Intelligence, ed. David J. Gunkel, (Edward Elgar Publishing, 2024), 13-26.
- Vergelijk de toepassing van VSAD in: Wynand Lambrechts, Saurabh Sinha and Sarah Mosoetsa, “Colonization by Algorithms in the Fourth Industrial Revolution,” IEEE (2022): 11057-11065.
- Ibo van de Poel, “Embedding Values in Artificial Intelligence (AI) Systems,” Minds and Machines 30, no. 3 (2020): 385-409
- See: Abeba Birhane, et al., “Power to the People? Opportunities and Challenges for Participatory AI,” EAAMO '22: Proceedings of the 2nd ACM Conference on Equity and Access in Algorithms, Mechanisms, and Optimization (2022): 1-8.
- Marc Steen, “Ethische aspecten bij het ontwikkelen en toepassen van AI. Een methode voor reflectie en de-liberatie,” Justitiële verkenningen 50, no. 1 (2024): 109-126.
Related Insights
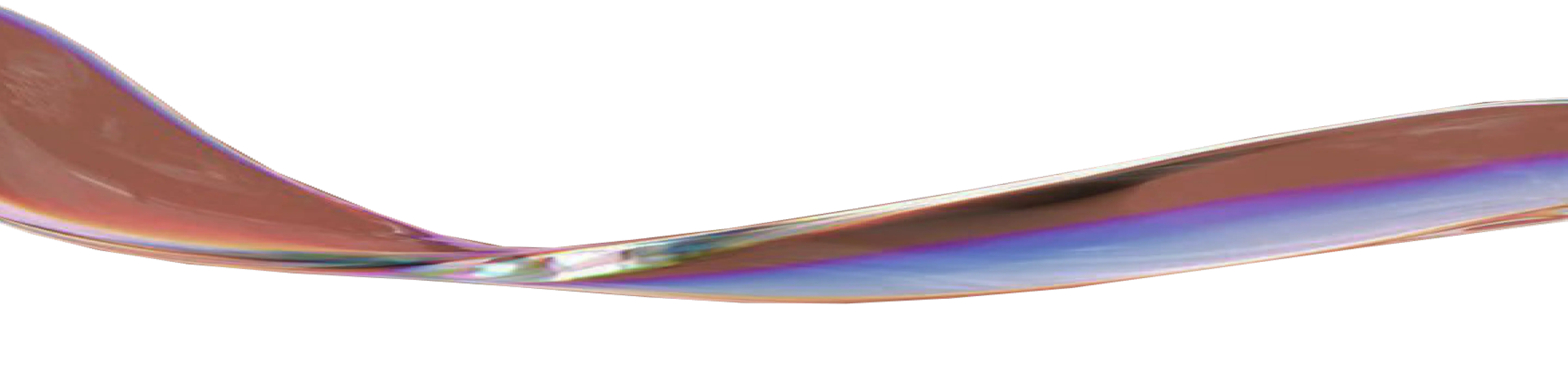