In control of your AI systems
This text is translated from Dutch with the help of AI - Have you ever heard or said within your organization, "We're working with data/algorithms/AI" or "We have plenty of data initiatives, but scaling within the organization is challenging"? These questions all boil down to one main question: How do you ensure that you apply the right algorithms in the right way?
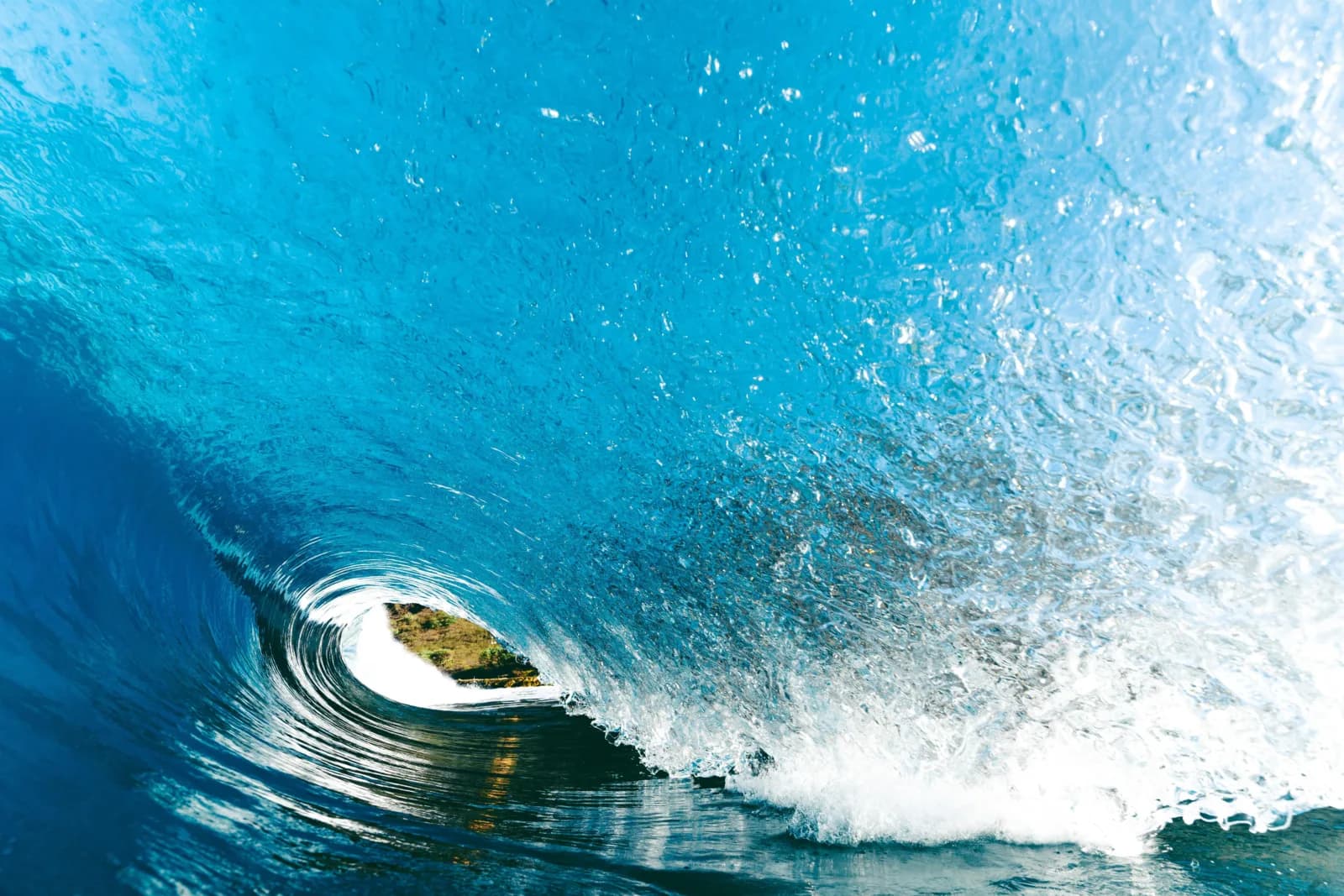
Criteria for responsible deployment of AI systems
The quality of an algorithm isn't solely determined by hiring brilliant minds to work on accurate algorithms. Experience teaches us that there's much more to it. Have you considered the following criteria?
- What should an algorithm fulfill to be considered 'good'?
- How do you establish the requirements for an algorithm?
- What acceptable margins of reliability exist when applying an algorithm?
These criteria play a crucial role even in the preparation stage to develop and manage algorithms and results of good quality, and also to achieve sound data quality.
Set explicit requirements
"Do you want to create an algorithm for me?" – algorithm developers need a more specific question than that. Picture you visit a hairdresser, you have a certain image in mind of what you want and especially what you don't want. Without explicitly stating your expectations beforehand, the hairdresser might cut your hair differently than you anticipated and you might walk out with the haircut of your nightmares.
The same applies to algorithms; they can't inherently meet the set of expectations without you being explicit. To meet your expectations, it helps algorithm developers to have answers to questions like: What problem do you want to solve using data? What data can, may, and should we use for this purpose? How do you intend to deploy the algorithm, and how does it align with existing business processes and current policies?
Reproducible and auditable results
Even when successfully developing and scaling an algorithm, we've observed that not every organization manages to ensure the algorithm continues to serve its intended purpose. In other words, how do you periodically evaluate that the algorithm still performs its intended function? To maintain control over managed algorithms, it's crucial to start with a deep understanding: begin with periodic checks on the algorithm's performance. Document what needs to be done if the check reveals that the algorithm isn't functioning as agreed upon. Don't just focus on the performance itself, but continually ask questions: What leeway does an employee have to deviate from the algorithm? How do you prevent tunnel vision in self-learning models?
In practice, managing algorithms is comparable to managing IT applications. Just like in applications, a process is executed based on specific input to achieve a desired outcome. Periodic assessments of application performance in terms of quality, speed, stability, security, and robustness are more the norm than an exception. This practice isn't only desirable to maintain control over algorithms, but might even become a requirement for algorithms, especially considering the upcoming obligations in the AI Act.
Periodic evaluation and improvement without excessive overhead
No matter how carefully an algorithm is developed, alignment between policy and execution is crucial for responsible usage. More and more organizations possess the knowledge and expertise to independently develop algorithms in a meticulous manner. These organizations have a solid grasp of how data flows through the algorithm.
Developers understand the choices made and assumptions taken. Lastly, they're aware of the impact of the utilized data and choices on the outcome. Similar to skilled hairdressers, these algorithm developers know how to achieve the desired end result.
However, achieving this desired outcome necessitates guidance for developers. On one hand, the connection between policy and execution requires ample understanding of algorithm usage within the management layer. Managers must be capable of setting frameworks and asking critical questions together with the execution team.
On the other hand, this demands a profound comprehension of data context and assumptions for developers. Just as you expect your hairdresser to advise you if your desired new hairstyle requires high maintenance or when it doesn't suit your face shape...
Central overview: AI registry
And what if you've already begun without clear instructions, but still want to remain in control? Often, there's no centralized overview of which algorithms are being used, and if such an overview exists, the documentation method varies across departments or even algorithms. How do you determine which algorithms are already in use in your organization and what their purposes are? To minimize the step towards transparency and accountability, having a single overview is helpful. Alongside this overview, a clear governance structure and management system regarding active AI systems is a prerequisite: who's responsible, for what, and when? Additionally, who can decide which changes will be implemented?
Where can I start today?
Does this sound familiar to you or your work environment? Would you like to be transparent about algorithms in use and be able to account for those?
- Establish a clear and actionable governance and management framework for the development, use, evaluation and control on algorithms and AI.
- Evaluate your algorithms at least similarly to how you should do with information security.
- Involve the right expertise throughout the entire AI lifecycle – from development to deployment – across all layers of your organization.
- Enhance transparency and accountability by implementing a central AI registry.
Gerelateerde insights
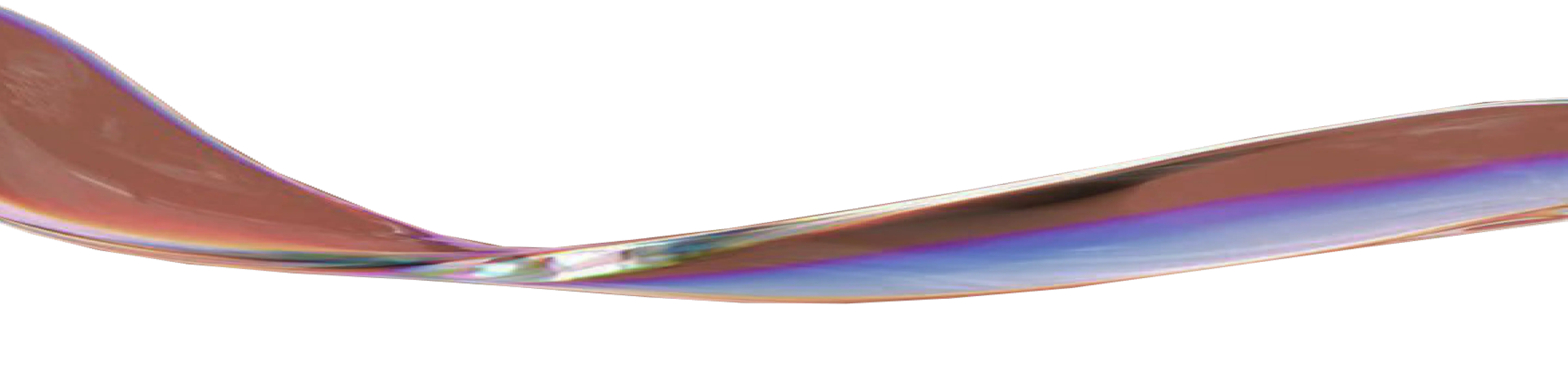