Data & analytics: Beyond the pilot phase
On February 3rd 2023, we organized a Round Table on Data & Analytics for civil society organisations. Large and small municipalities, provinces, ministries and the House of Representatives joined the virtual table. The central question that was at the table: How do we get from pilot to upscaling?
Many organizations have set up innovation teams or data labs. After initial successes, it often proves to be a challenge to ensure that data applications are well embedded in the organization, are scalable and are developed further in order to actually realize the added value of the new data application for the business. In this roundtable, we presented four dilemmas and discussed success factors for technical and organizational upscaling. With some additions from our side, we give a view of the afternoon in this insight.
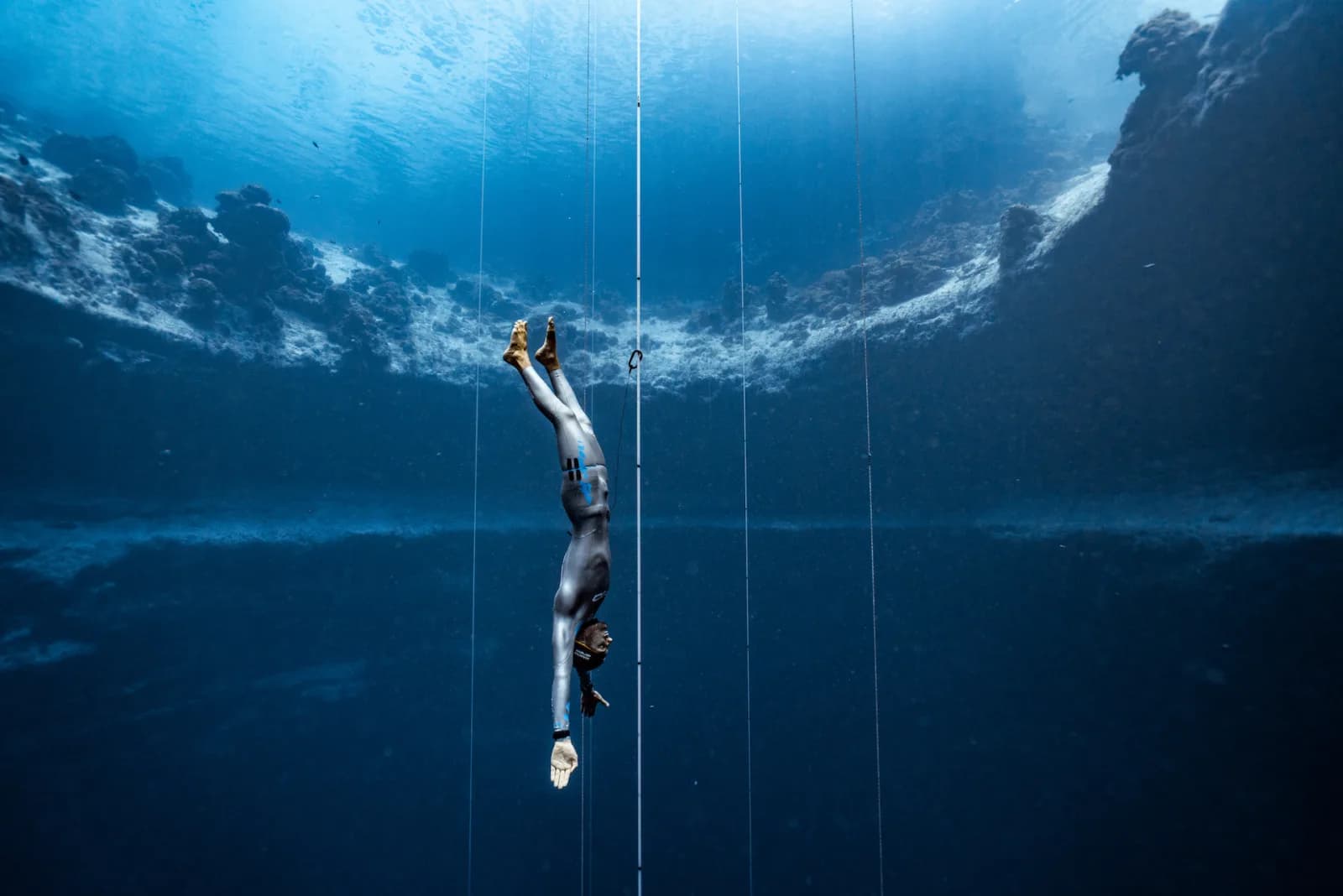
Dilemma 1 – The biggest challenge lies in: Technology or organization
Participants were reasonably in agreement about this dilemma. The technical possibilities can be overwhelming, especially in the beginning. However, renewal of the 'data foundation' is important for scaled use and it is sometimes difficult to get this right on the agenda. If we bring the right experts on board – a challenge in itself in the tight labor market – we will gradually gain a better grip on this. But in the end, the biggest job is to get the organization moving. Change is hard for people. How am I going to do my job differently? Do I want that? Scaling up a data solution often means that various employees in a process chain have to take on other activities, such as dashboard analysis and data management. Activities that must be drawn up concretely and explainably. And if someone does not take up their role, things can stagnate. Think, for example, of a product owner who enthusiastically brings his new dashboard to a driver, but who continues to steer it based on gut feeling.
Dilemma 2 – Collaboration of civil society organizations: As much as possible, in specific areas or limited
Most of the Round Table participants assessed cooperation in specific areas. Civil society organizations such as municipalities have joint tasks that can be strengthened with data-driven working. Cooperation in the development of this is interesting. But cooperation can also have a delaying effect. The wise thing to do is to take a good look at areas that can be accelerated by collaboration, beneficial and is even possible at all. For example, knowledge sharing on data-driven working in a use case such as youth care or sustainability is useful. But putting data in order is something that an organization will have to do itself. For some problems, data must be shared, and cooperation then provides an incentive to put your own data in order. Incorporating best practices can also be helpful. In any case, the following applies: Set up the collaboration in a targeted way and work towards interchangeable 'building blocks'. This way we ensure that the wheel has to be replaced less often.
Dilemma 3 – Innovation team: Development and/or implementation
A diverse picture emerges here. On the one hand, it is good to quickly take future implementation into account. In this way, user tests provide valuable information that further improves the product. Consequently, implementation requires specific knowledge and skills, such as domain knowledge, training skills and attention by management. In any case, let an innovation team focus on development and also consider whether the team has implementation skills, can strengthen these skills or can use reinforcement.
Dilemma 4 – The biggest challenge lies in: Demand articulation, development or scale-up
Not surprisingly, participants experience scaling up as the biggest challenge. The technical and organizational embedding of a data solution is more complex than running a pilot. It takes the most time, resources and perseverance. Often there is still no clear process for scaling up and it is gradually discovered what is needed for scaling up, hence costing learning money. Good demand articulation – upfront and during the development process – promotes smooth upscaling. Demand articulation is an art in itself: Who has what needs, how do you translate these into concrete functionalities and what preconditions for process, technology, data and organization are required for this? But when you get a clear picture of this, you can set preconditions for scaling up during the development process: such as data architecture, a DPIA and initial security measures and the right number of employees involved. Doing this right, you will be faced with fewer surprises and delays during scaling up.
Success factors
After a short coffee break, the group filled a digital board mentioning success factors for upscaling after a pilot. Success factors that may have recently emerged in their own organization or that were a translation of lessons learned and that organizations will focus on in the near future. As VKA, we supplemented the board with our practical experiences. In summary, this produces a varied and useful list:
- Connection with social task: Anyone can come up with an idea for a new data solution. But if we want to bring this data solution to sustainable development, it is good to make explicit how it contributes to a social task of the organization. This provides the necessary support and active participation from the line
- Enthusiasm: Enthusiasm makes the difference to continue developing and scaling up. With that you build the 'coalition of the willing' and you get through setbacks. Enthusiasm from not only a business owner or product owner, but all key roles involved within the organization.
- Management support: Ensure support and active involvement of management. Management support ensures that the data solution retains priority.
- Delivering value: Nothing is more convincing than proven value. So create value quickly and regularly to gain support for scaling up.
- Make success visible: Pay sufficient attention to communication for support, with user success stories via multiple channels and appealing demonstrations.
- An agile realization process: Define a process from demand articulation to development and scaling up, in which attention is paid step-by-step and 'neither too soon nor too late' to preconditions such as data management. This prevents delays during scaling up.
- Multifunctional team: Make sure that all permanent and temporary roles are represented in a multifunctional collaboration, in order to fulfill all preconditions for upscaling together.
- Roadmap: Make a global roadmap for business and IT. A roadmap makes it clear that the development and implementation of the data solution has several phases and a time investment, and it can be discussed which functionality is required in a pilot or scale-up phase.
- Professional knowledge: Also referred to as ‘data literacy’. Scaling up requires that everyone involved plays his/her role and that requires an investment in knowledge. Think of joint knowledge about the 'why' and 'how' of realizing data solutions at the organization in question and specific knowledge per expertise, from self-service analytics to data management, security and ethics.
- Knowledge sharing about working methods: When teams share reusable knowledge and experience about working methods, new data solutions can be scaled up faster.
- Data management: Formulate clear roles and agreements to ensure that the right data flows adequately from source to insight in the upscaling phase.
- Data architecture: This offers a great opportunity for upscaling within and outside the organization. By working with the principles of Common Ground, Open source and, for example, GEMMA (reference architecture for Dutch municipalities) and by gradually designing and working with the data architecture during the realization process, data can flow smoothly and reusable in the upscaling process.
To complete...
There is no such thing as 1 silver bullet approach for scaling up in the world of Data & Analytics, as we saw in the conclusion of the Round Table. It all depends... It is precisely the interplay of the factors mentioned earlier in this insight that ensures successful upscaling. An interesting observation was that many success factors are recognizable from the world of IT. Perhaps we can say that scaling up is a generic issue that is of all times. In the field of Data & Analytics, two new factors will be added: Data management and data architecture. Not easy to set up, but decisive for upscaling and the degree of reuse. To point out one silver bullet: finally, one of the participants emphasized enthusiasm as a success factor for upscaling. As VKA we also have this experience: Where there is a lot of positive energy and drive, more success factors follow!
Related insights
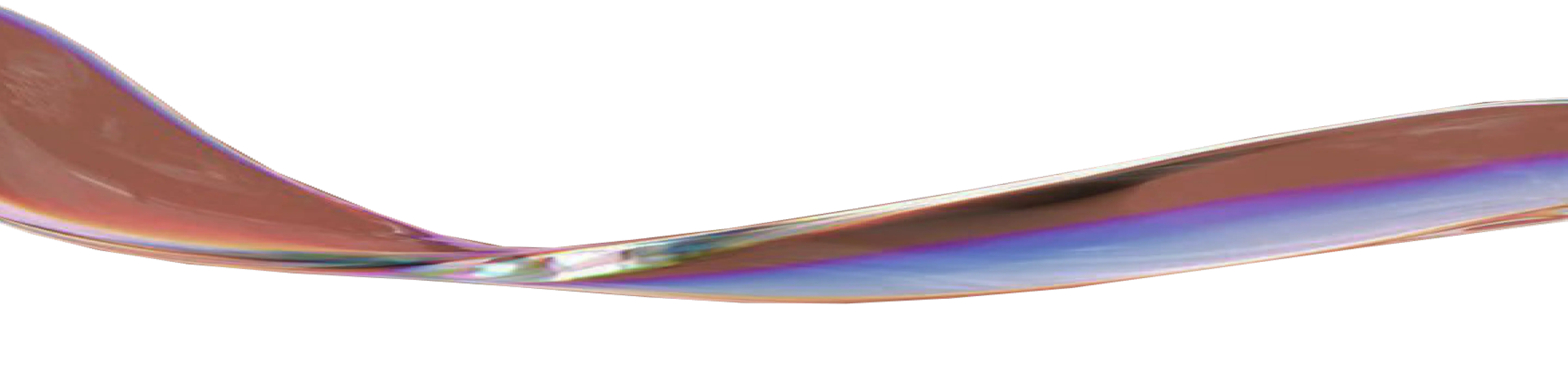