Algorithm transparency saves lives
Who does not use them: Alexa, Google Assistant or Siri? Smart voice recognition apps with intelligent software that learn from your questions and therefore come up with better and better answers. Unfortunately, the answers now are sometimes awkward, ignorant or even wrong. For a simple question to turn on the radio AND take out commercial breaks, she does not come out even after many repetitive questions. Why, how and what? Really no idea because it is not made transparent. This is no big deal for funny applications but for serious ones, a mistake can cost lives or mean social discontent.
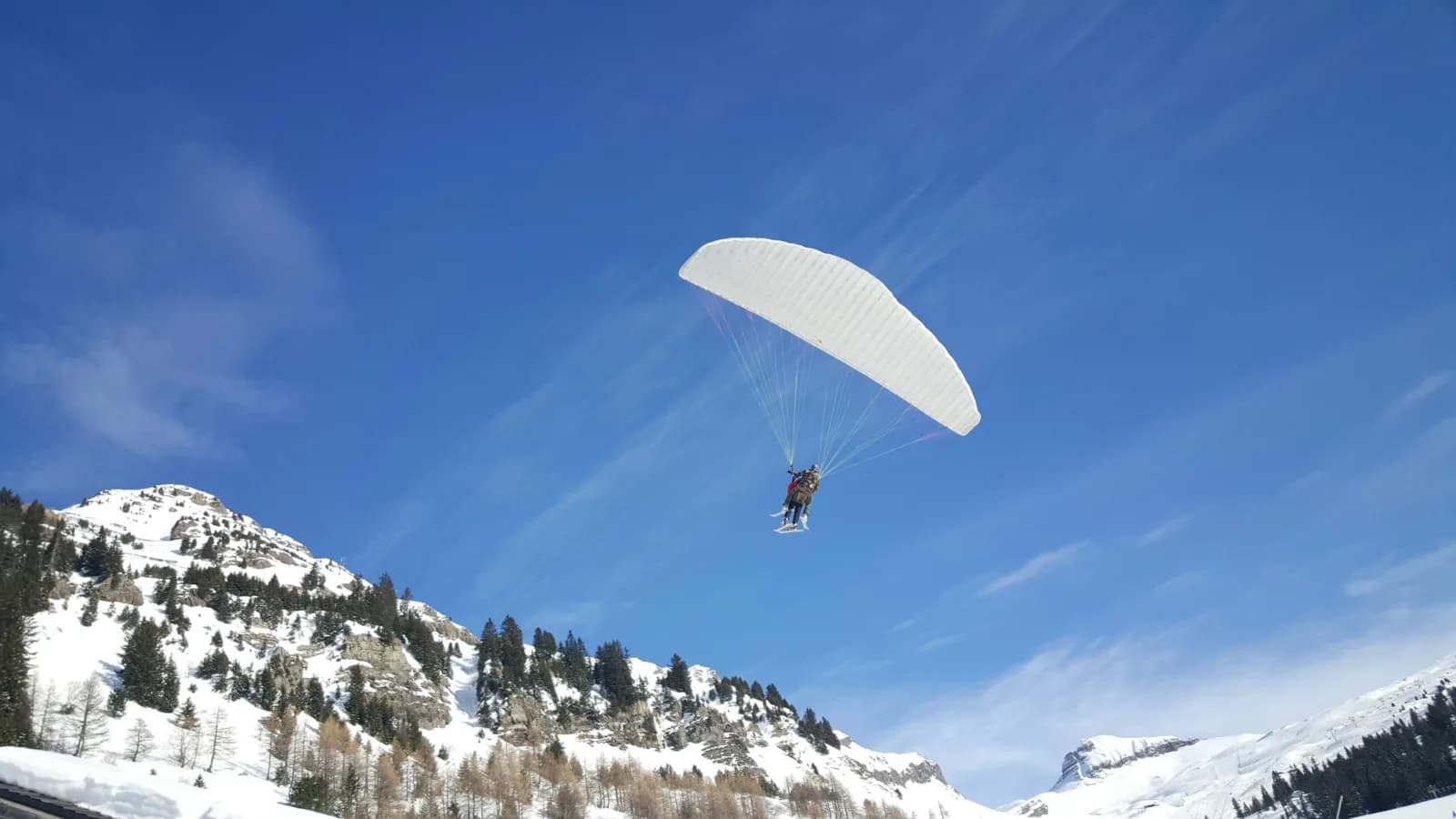
A well-known example is the Amazon 'AI application for recruitment' that did not assess gender neutrality for recruitment. The algorithm was found to have preferences for male experts.
Another shocking example is that of Uber's autonomous driving car. Uber had developed an algorithm-equipped autonomous driving car that failed to intervene in time when crossing a pedestrian resulting in death. A major cause of the accident was an algorithm that, due to late and vague inputs from sensors, decided to continue driving because braking was pointless while swerve at the expense of the car was also not an option. Algorithms by definition, like human work, are not always objective. An algorithm test and laboratory trial before the Uber car hit the road could have prevented the worst.
Since the advent of artificial intelligence (AI), complexity is increasing and reliability is a growing challenge. Intelligence is created by using algorithms. These algorithms are written by software specialists. The writing is human work, where the algorithm is difficult to make transparent and objective as in the example of Uber autonomous driving and gender-neutral recruitment at Amazon.
Therefore, it is necessary to have the algorithm independently tested. Testing makes the quality of the algorithm transparent and makes it explainable towards users and regulators. An algorithm test according to VKA is a review to what extent your algorithm meets generic principles such as: conscious use, based on knowledge of, privacy by design, learning, controlled application, transparent, and social explainability.
Here are the top three tips to increase the governability, transparency, predictability and supportability of AI and the algorithm.
Tip 1. Test algorithms independently.
If Uber had done an independent algorithm test and lab trial before hitting the streets in 2018, it would have saved a life. By the way, you will have to keep testing and testing algorithms even after implementation. In Amazon's case, the "bias" could have been avoided by using an independent algorithm test. Such a test looks not only at generic principles as described above, but also at the degree of data quality, ethics and subjectivity in practices.
Tip 2. Check your data quality.
It is important to know the quality of your data, especially if you combine "data lakes". Are there privacy data or gender data that you want to delete before using the data? Is the data good enough for use or are unreliable data blocks identifiable? Prepare the data for use. Check to what extent historical data are still representative of desired or future outcomes. Cleaning up or removing data prevents subjectivity or "bias".
Tip 3. Make the algorithm transparent so that it is socially explainable.
Whatever the goal, however the software specialist has proceeded, however well it seems to be regulated around privacy, however complex it is, ask the question: "are benefits and drawbacks explainable to all stakeholders?". Ask for transparency on (cleansed) data, algorithm operation and work processes. Do the tested outcomes have a possible impact on public support? Know the "bias" of the algorithm and always communicate the possible social - advantages as well as disadvantages. That way it is socially explainable.
A powerful possible "live saving" example of the use of algorithms is from earlier this year. The Deepmind Google algorithm recognised breast cancer better than the human doctor. Unfortunately, there were also complaints about transparency of (privacy) data agreements. With an independent algorithm test, Google might have avoided this hassle. An algorithm test on with transparency in the "hey google" algorithm could have resolved my ignorance or improved my understanding.
My tip to Google: 'Algorithm transparency saves lives and may solve my ignorence'.
Related insights
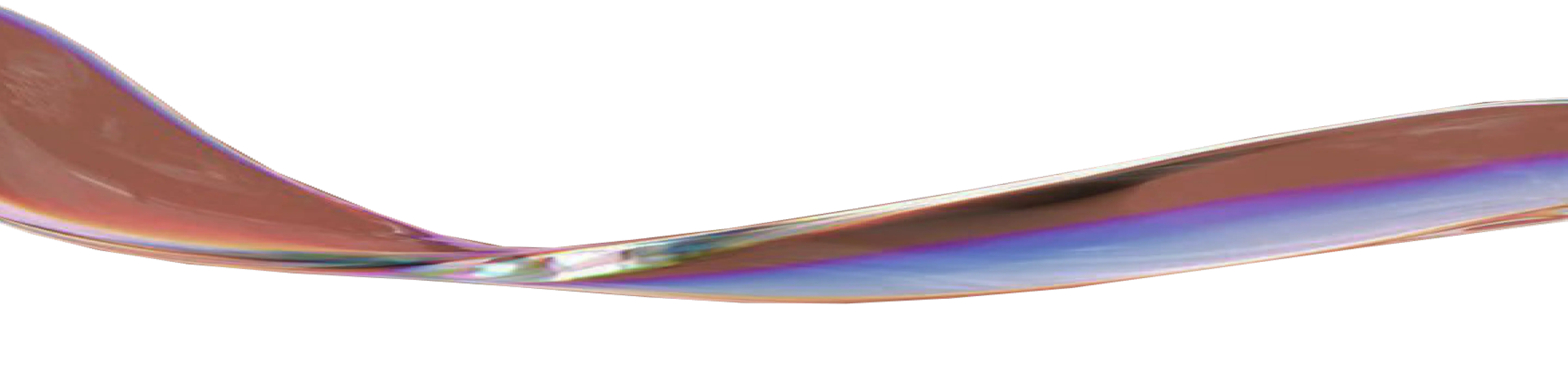