4 tips to prevent discrimination by algorithms
No discussion about algorithms goes by without the word 'discrimination' being mentioned. Understandably so, as there are now numerous unfortunate examples where people suffer greatly from decisions made based on algorithms. And this harm sometimes goes so far as to disrupt or destroy lives. That is, of course, unacceptable – but how can you maintain control over discrimination by algorithms?
The bad news: discrimination is inherently linked to algorithms. Why? The original meaning of discrimination comes from Latin. To discriminate means to 'distinguish.' Only later was another meaning added to the word 'discrimination,' namely that someone is treated unequally based on discrimination.
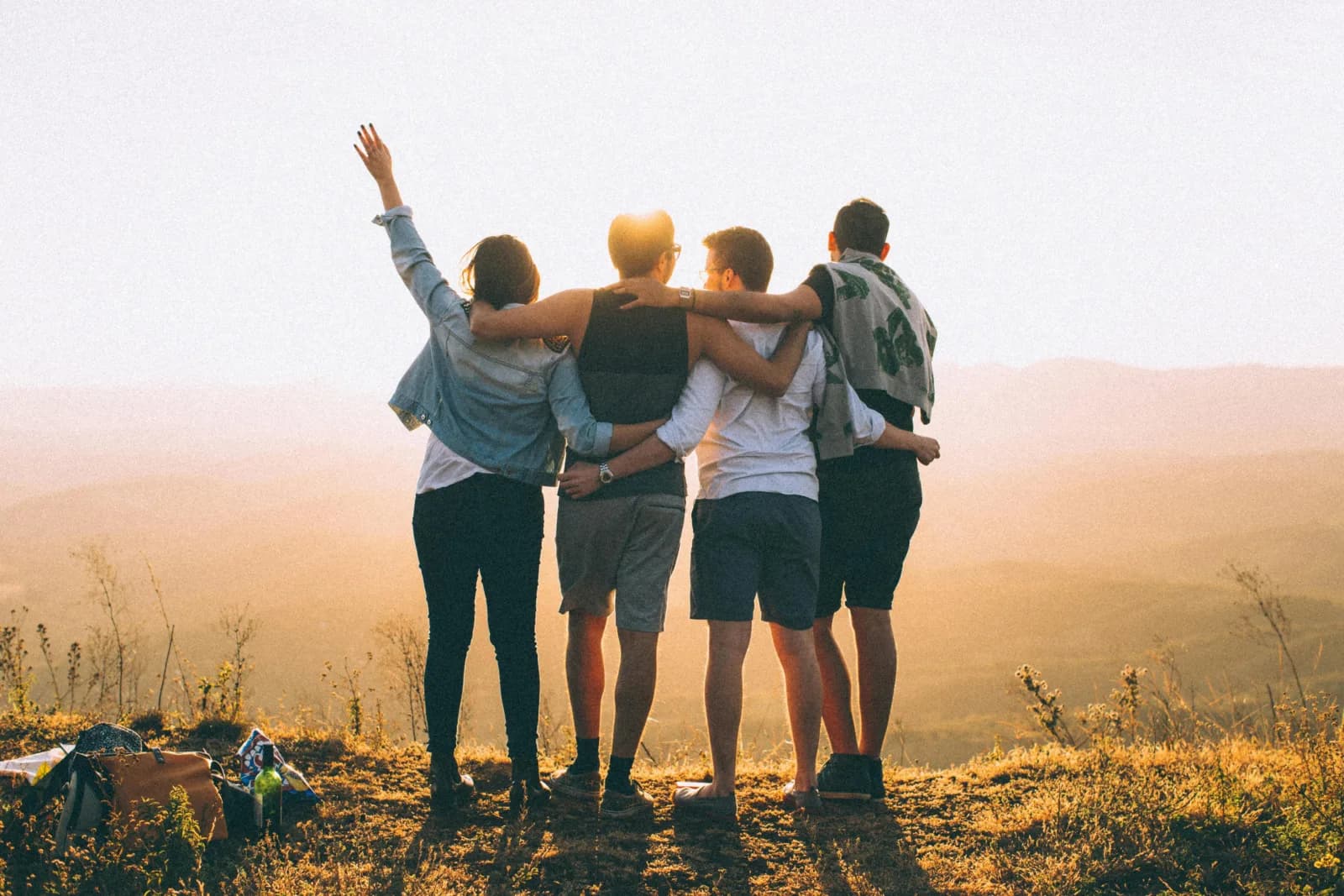
To me, this also highlights the issue of discrimination by algorithms: algorithms are adept at distinguishing 'groups' based on characteristics. Discrimination arises here as well when a group is treated differently because the outcome of the algorithm is adopted without suitable assessment or counterargument without human intervention.
I encounter many clients (companies, enforcement agencies, and municipalities) that use algorithms. The risk of discrimination is particularly prominent in departments dealing with fraud prevention and security research. Why? Because they must be able to distinguish those who don't behave in the large group of people who do, in order to address them. And if they can't identify these 'bad apples,' the consequences can be significant (think of increased crime, loss of money due to fraud). Algorithms are developed in these places to identify the bad apples. As a result, individuals distinguished by the algorithm from others automatically carry an aura of being a 'bad apple.' In such situations, you can prevent algorithms from discriminating in various ways:
1. Decouple the algorithm's outcome from the subsequent action. Suppose you've developed an algorithm that signals potential fraud. Ensure that a payment is not automatically halted but subject to human evaluation.
2. Ensure that human assessment is unbiased. I've seen many examples where there was human evaluation, but it wasn't impartial. Sometimes, due to workload, blind trust is placed in the indication given by an algorithm ('red and green' or, through naming, 'indication of fraud').
3. Many algorithms are developed to identify negative behavior and further investigate anything negative. These algorithms are designed to predict, with the highest possible level of reliability, whether someone is committing fraud or poses a security risk. However, this can be reversed: develop algorithms to rule out that someone poses any risk, at the very least. Look for variables that demonstrate there's nothing wrong. An additional advantage is that you won't unnecessarily allocate scarce investigative resources and won't burden people who are likely to behave properly with unnecessary additional questions or investigations.
4. Algorithms don't exist in isolation. They are a tool in a process of (for example) fraud prevention and crime-fighting. Algorithms are often constructed based on knowledge contributed by real, flesh-and-blood people. In developing algorithms, it's also necessary to continually assess the objectivity of the human knowledge. This means that there should always be a control group that isn't assigned to a group by algorithms. Ensure that this is done by ordinary people who make choices based on their expertise. You can then periodically compare these choices with your algorithm to determine if the algorithm is still making the correct judgments, just as 'real people' do.
By implementing these 4 tips in practice, you can both benefit from the advantages of algorithms and prevent the disadvantages. Good luck!
Related insights
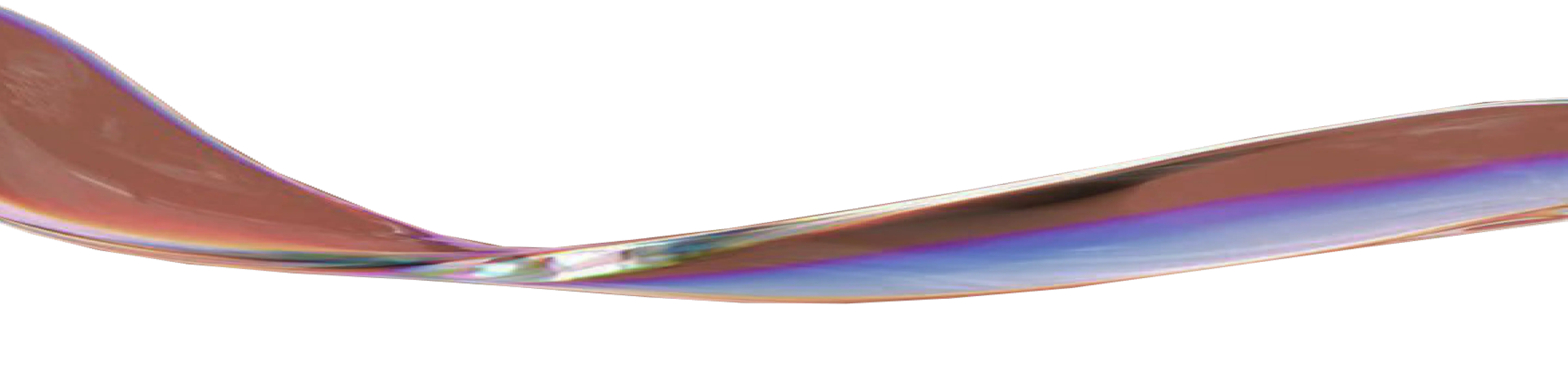